An annotated image dataset of pests on different coloured sticky traps acquired with different imaging devices
New publication by Song Quan Ong & Toke Thomas Høye.
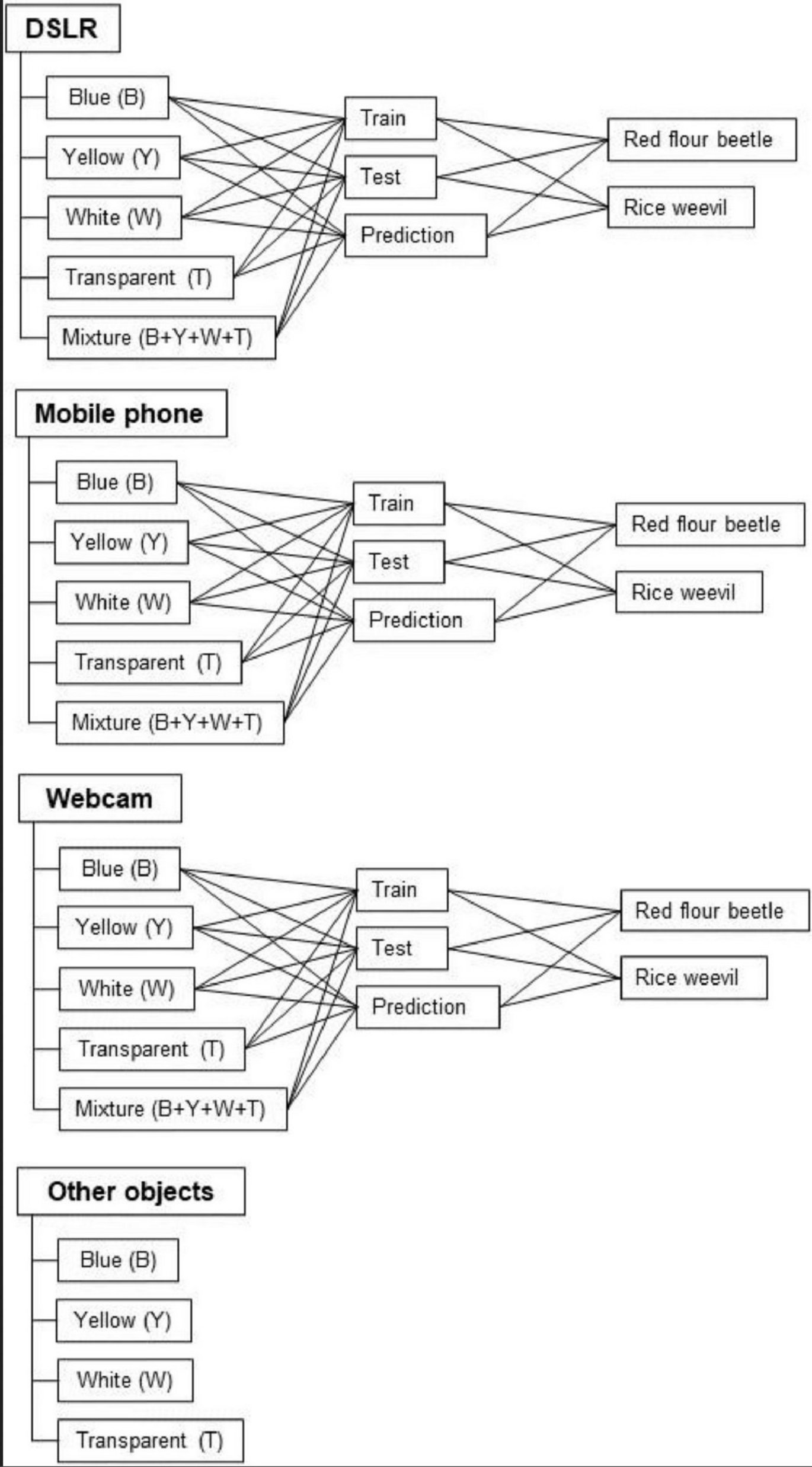
Abstract:
The sticky trap is probably the most cost-effective tool for catching insect pests, but the identification and counting of insects on sticky traps is very labour-intensive. When investigating the automatic identification and counting of pests on sticky traps using computer vision and machine learning, two aspects can strongly influence the performance of the model – the colour of the sticky trap and the device used to capture the images of the pests on the sticky trap. As far as we know, there are no available image datasets to study these two aspects in computer vision and deep learning algorithms. Therefore, this paper presents a new dataset consisting of images of two pests commonly found in post-harvest crops – the red flour beetle (Tribolium castaneum) and the rice weevil (Sitophilus oryzae) – captured with three different devices (DSLR, webcam and smartphone) on blue, yellow, white and transparent sticky traps. The images were sorted by device, colour and species and divided into training, validation and test parts for the development of the deep learning model.