Effects of waste-based pyrolysis as heating source: Meta-analyze of char yield and machine learning analysis
New publication by Zhenhua Huang, Maurizio Manzo, Changlei Xia et al.
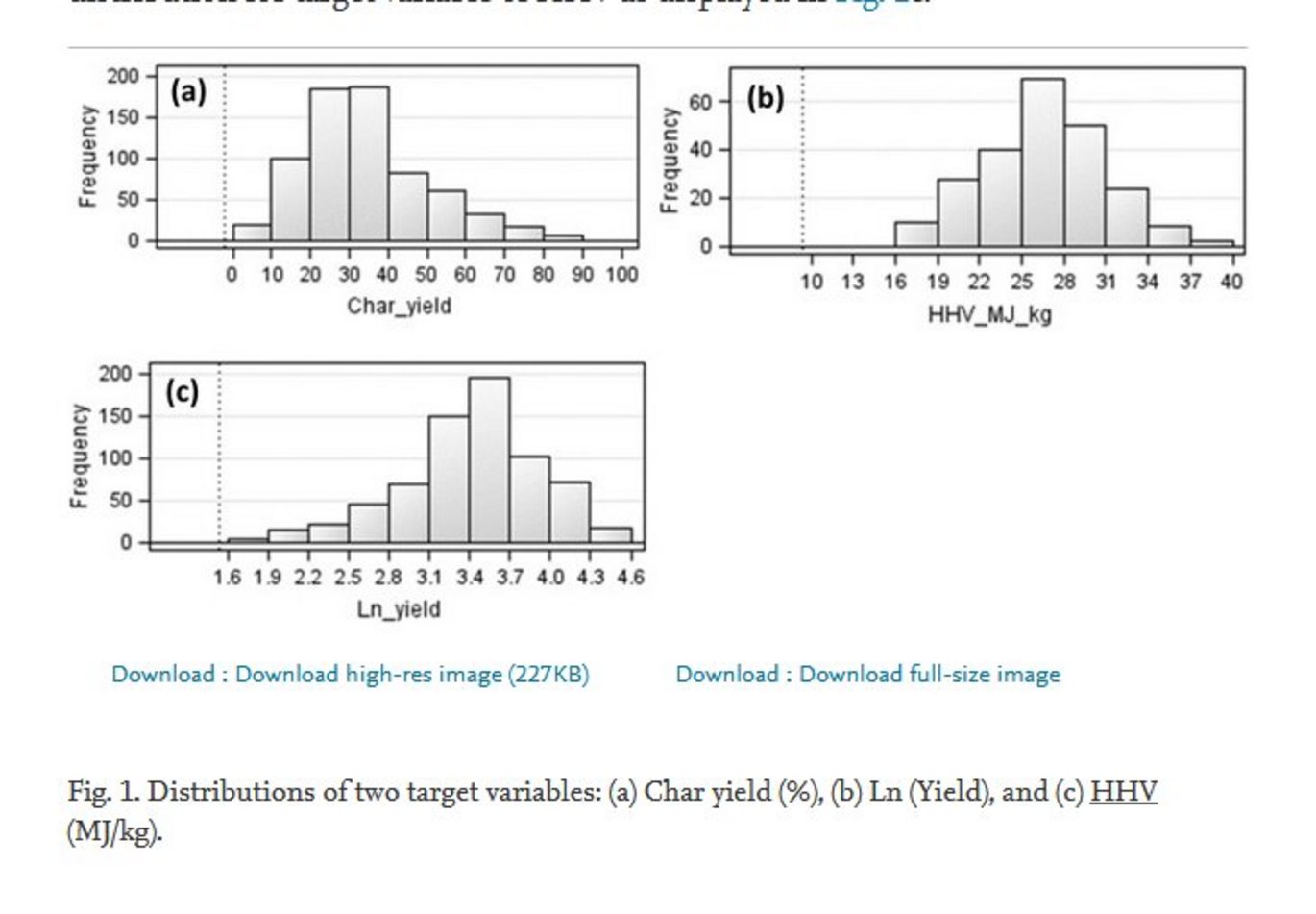
Abstract:
Among three energy products of oil, char, and gas from pyrolysis, char has the advantages of easy operation and low cost. It is expected that pyrolysis of waste materials using a desired heating source and optimal operation parameters can achieve max pyrolysis char yield with good higher heating value (HHV). Here we evaluate the effects of four heating sources being direct thermal, microwave radiation, solar, and infrared heating, and the operation parameters involved, i.e., heating rate, final temperature, dwelling time, and feedstock type and size, on pyrolysis char yield and HHV. We collected 61 pyrolysis cases with 683 individual observations from the international scientific literature and used the big data in machine learning (ML) analyses. The target variables were char yield and HHV, while the input variables were heating source, feedstock type, feedstock size, temperature, heat rate, and dwelling time. Five ML technologies, including Linear & polynomial regressions, k-Nearest Neighbor (kNN), Artificial neural networks (ANN), and Gradient boosting were performed. The decision tree results indicated that the relative significances of input variables to the target variable of char HHV decreased in the following order: feedstock type > heating source > particle size > heat rate > dwelling time > temperature. While the relative significances of the input variables to the target variable of Ln (char yield) decreased as: temperature > heat rate > dwelling time > particle size > heating source > feedstock type. The Ln (char yield) and char HHV (MJ/kg) can be accurately predicted based on known input variables using the developed Polynomial regressions. After comparing the five ML prediction models, we conclude that the Gradient boosting model is the best to estimated Ln (char yield) and HHV with high accuracy, which can be used to predict Ln (char yield) and HHV based on the type of pyrolysis feedstock and operation parameters.