Hierarchical classification of insects with multitask learning and anomaly detection
New publication by Bjerge K, Geissmann Q, Alison J, Mann HMR, Høye TT, Dyrmann M et al.
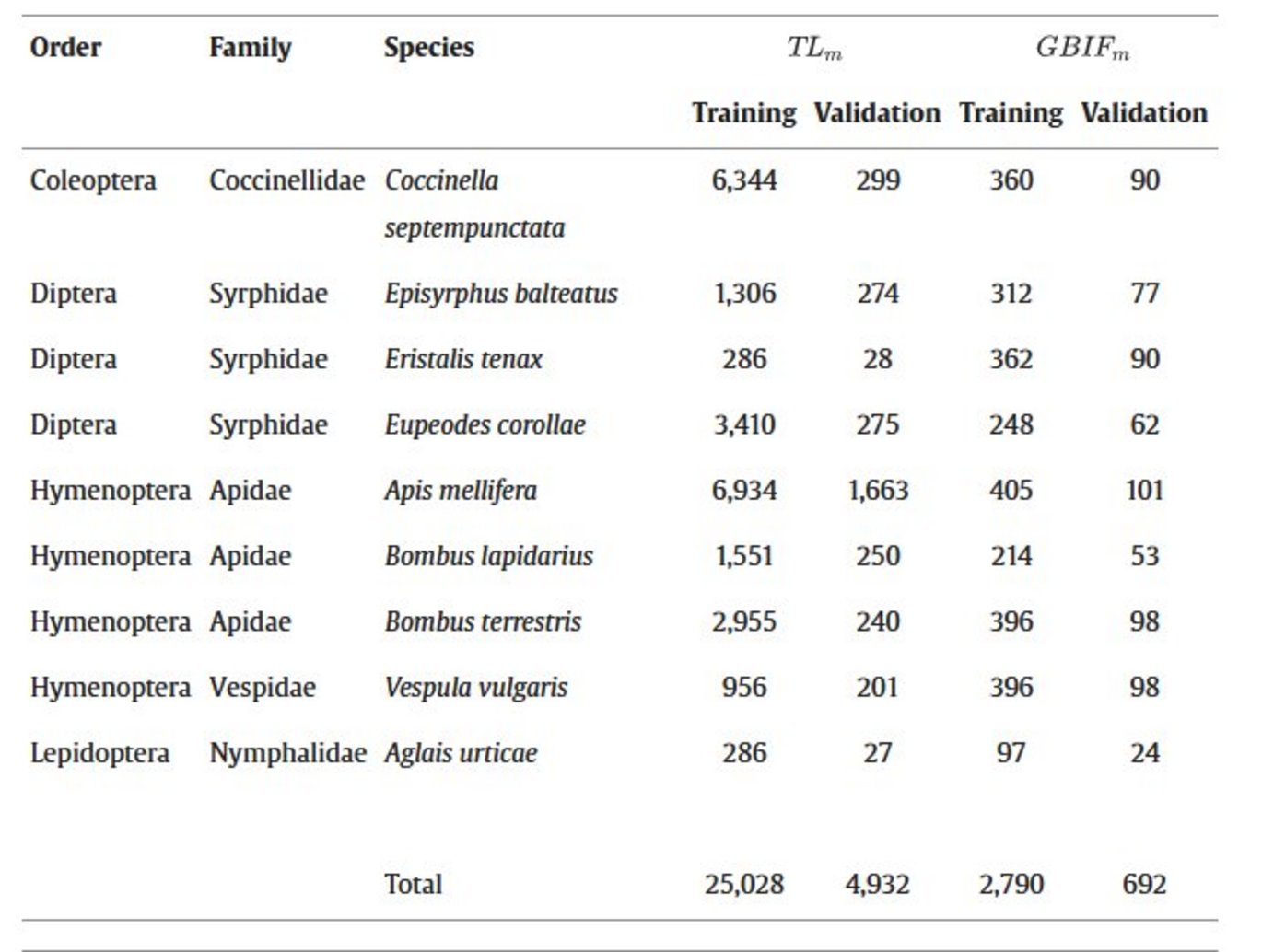
Abstracts:
Cameras and computer vision are revolutionising the study of insects, creating new research opportunities within agriculture, epidemiology, evolution, ecology and monitoring of biodiversity. However, the diversity of insects and close resemblances of many species are a major challenge for image-based species-level classification. Here, we present an algorithm to hierarchically classify insects from images, leveraging a simple taxonomy to (1) classify specimens across multiple taxonomic ranks simultaneously, and (2) identify the lowest rank at which a reliable classification can be reached. Specifically, we propose multitask learning, a loss function incorporating class dependency at each taxonomic rank, and anomaly detection based on outlier analysis to quantify the uncertainty. First, we compile a dataset of 41,731 images of insects, combining images from time-lapse monitoring of floral scenes with images from the Global Biodiversity Information Facility (GBIF). Second, we adapt state-of-the-art convolutional neural networks, ResNet and EfficientNet, for the hierarchical classification of insects belonging to three orders, five families and nine species. Third, we assess model generalization for 11 species unseen by the trained models. Here, anomaly detection is used to predict the higher rank of the species which were not present in the training set. We found that incorporating a simple taxonomy into our model increased the accuracy at higher taxonomic ranks. As expected, our algorithm correctly classified new insect species at higher taxonomic ranks, while classification was uncertain at lower taxonomic ranks. Anomaly detection can effectively flag novel taxa that are visually distinct from species in the training data. However, five novel taxa were consistently mistaken for visually similar species in the training data. Above all, we have demonstrated a practical approach to hierarchical classification based on species taxonomy and uncertainty during automated in situ monitoring of live insects. Our method is simple and versatile, forming a valuable step towards high-level classification of species not found in training data.